2 State of the art
The state-of-the-art analysis in this study explores key aspects of energy optimisation at the district scale. This chapter highlight various methods for estimating buildings’ energy demand, the concept of pooling of energy balance within energy communities, and the role of community energy storage in enhancing energy production and consumption. By synthesizing insights from the literature, this review contributes to our understanding of building energy demand at district scale and offers valuable perspectives for future research and implementation.
2.1 Methods for estimating buildings energy demand at district scale
Energy optimisation used to focus on individual buildings, considering their energy demand and efficiency. The utilization of the energy hub model enables the evaluation and optimisation of energy flow management (Orehounig et al. 2014). This concept can be applied either at one building level or at a district level depending on the research. In their work, Fonseca and Schlueter (2015) propose a shift from the classical description, namely quantity and quality, of energy demand to a spatial and temporal description. In addition to proposing this change at the level of description, they do not work with a micro level of analysis, namely a building, but with a macro level of analysis, namely a group of buildings. The objective of their paper is thus to obtain spatio-temporal patterns of energy consumption at the neighborhood level. Optimising energy at the district level through an energy hub enables the aggregation and coordination of diverse energy sources and demands within the district. In the literature, two opposite building stock modelling approaches are used: the top-down and the bottom-up (Kazas, Fabrizio, and Perino 2017; Swan and Ugursal 2009).
- The approach of top-down modelling involves the study of the building stock as a whole, without focusing on individual building models, technologies or energy end-uses. This type of modelling was developed to investigate the energy consumption of buildings and to link it with long-term changes in the building sector, such as historical, economic, and technological changes. It is based on data only. Top-down models rely on readily available aggregated data, such as gross domestic product (GDP), climate parameters, construction parameters, and estimates of the number and usage of appliances. Top-down modelling approaches can be classified into two categories, which are econometric and technological models. They are based on the type of input data they use.
Econometric models assess the energy consumption related to economic and macroeconomic parameters, such as fuel prices, income, gross domestic product etc. The main drawback of econometric models is that they only focus on historical, economic and statistical data. This model do not take into account physical and technological factors or their evolutions. When there is a lack of information on the evolution of the technological factors, it is difficult to reliably predict the energy demand, energy consumption and \(CO_{2}\) emissions of the building stock, because the future environmental, technological and economic conditions may be different from those of the past.
Technological models evaluate the energy performance of a building stock by analyzing technological data like technological progress, along with architectural and structural modifications. Such models focus on technological factors at an aggregated level and primarily examine the national building stock. It is difficult to precisely predict the energy demand, because the technological top-down model parametrisation is not carried out at a detailed level.
- The bottom-up modelling approach is a detailed method that investigates the building stock by starting at a disaggregated level, using various aggregation methods and techniques to represent the entire building stock at an urban or national scale. This approach uses hierarchical data from the individual end-user, building, block of buildings, urban district, regional, or national building stock scale, allowing for energy demand prediction, demand-side management strategy development, and environmental issue evaluation and mitigation. Bottom-up models include data-driven, physical, and hybrid models and require extensive empirical data databases to develop and validate the models for reliable results. This approach can be classified into two sub-categories: statistical and engineering models.
- Statistical bottom-up models rely on historical and energy performance data to establish the connection between end-uses and energy consumption. These data-driven models can approximate the energy consumption of a representative group of buildings, ranging from a neighbourhood to a city or national scale. Statistical techniques like regression allow the evaluation of the energy performance and estimate the energy consumption. However, the bottom-up statistical modelling approach does not provide comprehensive information on end-uses like energy consumption, heating and cooling applications, or appliance use. Additionally, the reliability of statistical modelling requires a significant amount of data, which can be sourced from either public or private databases.
- Engineering or bottom-up physics-based building models utilize heat transfer and thermodynamic equations to assess energy performance, accounting for the use of appliances, equipment, and power ratings. These models are highly detailed and can evaluate the energy performance of each end-user. The bottom-up engineering approach involves clustering and classification techniques, including archetypes or samples of representative buildings.
In the field of energy demand analysis, a large number of approaches and methodologies have been proposed in scientific literature, leading to a considerable diversity of results and outcomes. A large literature review of over 70 studies has been conducted by Ferrari et al. (2019), aiming to classify the selected contributions based on the features of the energy demand data source and/or estimation methods. A summary scheme of adopted classification is represented in Figure 2.1.
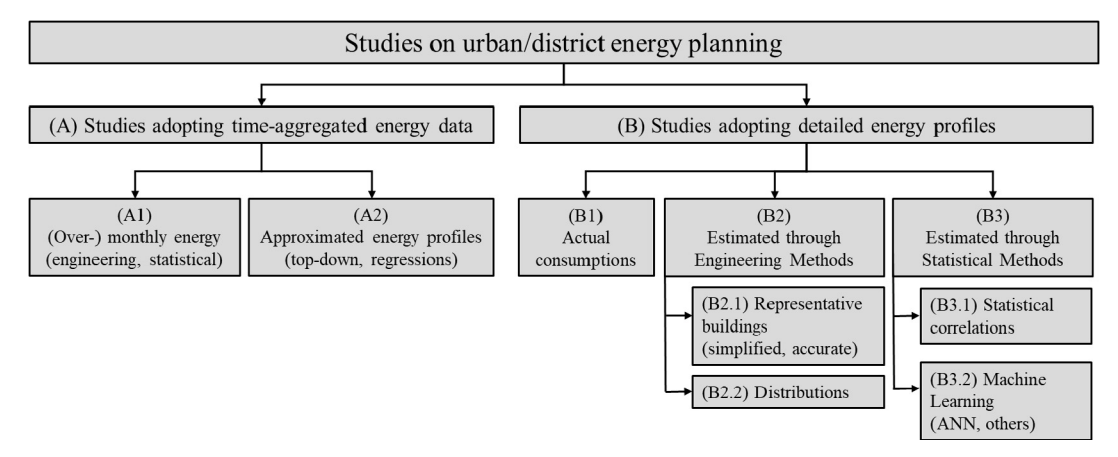
Figure 2.1: Scheme of adopted classification in the literature review made by Ferrari et al. (2019)
An explanatory table is presented below. It provides a brief explanation of each of the methods written in Figure 2.1.
Method | Explanation |
---|---|
Studies adopting monthly energy data (A1) | Some studies calculate annual or monthly energy demand using either engineering or statistical methods to assess the balance between potential energy supply and energy loads. However, this approach is only suitable for early-stage analysis and results in highly approximate outcomes that may differ significantly from more detailed results. |
Studies adopting approximated energy profiles (A2) | To obtain more precise energy assessments, certain studies have adopted techniques to derive approximate profiles from top-level data despite significant lack of data. These techniques include correlations with weather data and building use category or typology. |
Studies adopting actual energy consumption profiles (B1) | Obtaining actual hourly based energy consumption is ideal for accurately estimating energy fluxes in a case study. Hourly data can be obtained through monitoring campaigns in specific areas, such as university campuses, or can be provided by electricity distribution utilities or district heating networks. Mesured energy consumption profiles are used in this method. |
Studies adopting energy profiles estimated through engineering methods (B2) | Engineering methods, also referred to as physical or white-box methods, are based on the detailed analysis of physical laws governing the building’s thermal energy balance and electric appliance consumption. They are deterministic, meaning that given inputs result in certain outputs. These methods provide highly accurate estimations when based on dynamic energy simulation. However, when applied on an urban scale, the required level of detail and amount of information can be time-consuming, making detailed building energy simulations impractical. Simplifications are often adopted. |
Studies adopting hourly energy profiles estimated through statistical methods (B3) | Statistical methods, also known as black-box or data-driven methods, rely on correlations between inputs and outputs, which are derived by assigning weights and biases. Although statistical methods may require less detailed data than engineering methods, they are useful in dealing with data uncertainty, randomness, and scarcity, making them appropriate for predicting stochastic phenomena such as consumer behavior. Due to the growing popularity of statistical methods in building physics research and applications, there are now several techniques available as statistical correlations (B3.1) or machine Learning methods (B3.2). |
We have observed that energy optimisation at the district level is increasingly used in research for urban planning. Two methodological approaches exist, as well as different modeling approaches. In our work, we will adopt a bottom up approach and use modeling through engineering methods.
2.2 Pooling of energy balance in energy communities
Intense energy production at a time of low consumption or high demand at a time of low production leads to energy imbalance. Additionally, it is rare for production and consumption peaks to align perfectly. This disparity between energy surplus and deficit, whether related to production or consumption, poses a challenge that necessitates resource optimisation. An intuitive solution to address this challenge would be the compensation between the excess and the deficit. The ability of production and consumption to offset each other may be called pooling energy balance. The emergence of energy communities offers the possibility to implement this balancing approach. The mutualization of buildings within an energy community causes a stochastic effect due to the varying timing of consumption peaks, which induces a smoothing of the consumption curves, facilitating a more efficient use of energy resources.
Compensating energy production and consumption is a major challenge. Our interest is to do it at a district level. There is no consensus on how to express this idea of compensation. Indeed, different precise concepts are used depending on the subject in question. We decided to use the concept of pooling energy balance because it reflects both the idea of compensation and aggregation. The objective is to achieve a balance between energy surplus and deficit by leveraging the collective capacity and flexibility of energy consumers and producers within the system. To manage the fluctuating supply and demand dynamics, a geographic area must be defined. Energy communities are perfectly fitted to apply the pooling energy balance.
From a technical point of view, energy communities can be seen as a distributed energy system where multiple small-scale renewable energy installations are interconnected within a specific district or neighborhood (Kubli and Puranik 2023). These installations may be micro-hydropower systems, solar panels or wind turbines. They work, along with energy storage systems and demand-side management, to optimize the energy production and consumption, and the distribution within the community. The energy production and the consumption patterns are uncertain. Regarding the renewable energy sources, this randomness refers to the intermittency of the production. Regarding the consumption, it is due because all individuals of the energy community do not reach their peak of consumption at the same time, leading to uncertain demand which needs to be considered in order to ensure a reliable and stable energy supply. When the consumption of each individual of the community is aggregated, a stochastic effect may be observed. This effect implies that the consumption curve is smoother than in the case of individual consumption.
In addition to the environmental benefits that energy communities generate, there also are socio-economic benefits. Being part of a community allows citizens to participate in the energy transition and provides direct potential benefits such as creating local jobs, lowering their electricity bills, keeping money in the local economy and promoting local investment (European Parliament, Research Service 2020).
2.3 Community energy storage (CES)
The emergence of an energy community makes it possible to pool energy production and consumption in order to reduce the disparity between energy surplus and deficit. Sharing installations raises the question of energy storage. Indeed, introducing the idea of storage into the concept of energy communities makes it possible to go even further, not only in optimising the energy produced, but also in reducing costs.
Renewable energy sources suffer from intermittency. Wind is a perfect example: electricity production from wind is completely fluctuating and difficult to predict (Ibrahim, Ilinca, and Perron 2008). As a result, the idea of storing these energies for later use emerged. Storage becomes a key element for the growth of renewable energies (Ibrahim, Ilinca, and Perron 2008). Surplus energy obtained can be stored in several ways. Different classifications and characterisations exist. Hossain et al. (2020) propose the following classification: mechanical, electrochemical, chemical, thermal energy, as well as in the form of electric or magnetic fields. An hybrid form of storage based on electric and magnetic fields is also available. The advancement of energy storage requires consideration not only of the technologies employed, but also of the scale disparity between centralized systems and the growing prominence of decentralized systems, such as energy communities (Hernandez-Matheus et al. 2022).
The concept of community energy storage has emerged from this observation. Several definitions exist, but no consensus has yet emerged on a single one (Koirala, van Oost, and van der Windt 2018). By considering energy communities as a means to address the disparity between energy production and consumption, and incorporating the concept of storage, we propose to adopt the following definition for the community energy system as an energy storage systems located at the consumption level with the ability to perform multiple applications to manage demand and supply (van der Stelt, AlSkaif, and van Sark 2018). Consequently, community energy system can be seen as a compromise between a single-home energy system storage and grid-scale energy system storage in order to balance the generation of energy and the demand (Parra et al. 2017). The underlying idea also reflects the fact that replacing building-scale storage system with larger ones for the whole community is more cost-effective and efficient. Sharing installations offers direct and indirect economic benefits by reducing installation costs and promoting better management of supply and demand on the grid. Parra et al. (2017) also point out that the environmental benefits are significant, as this storage system enables greater penetration of renewable energies into the environment by:
- allowing end users to shift surplus generation to meet their demand load later
- maintaining grid stability
- internalising system benefits into economic revenues when taking part in different markets
- catalysing grassroots initiatives with the participation of community members that facilitate the socio-economic development of the district/community
Whatever the level at which energy storage is deployed, namely at a household or a community scale, the question of storage is crucial (Dong et al. 2020). Defining the energy hub as a unit where the production, consumption, storage and conversion of multiple energy carriers happens and considering contributions about the development of the storage at the level of a community, community energy storage may be viewed as an energy hub. An important element in analyzing hub energy is understanding the link between each prosumer making up the community and the community itself, so as to take into account each member’s consumption while optimising each individual’s production and storage system (Gu et al. 2023).