2 State of the art
2.1 Energy planning and modeling
Although the focus of planning has primarily been at the national level, prior studies (Bhatt, Friley, and Lee 2010) have highlighted the importance of adopting integrated strategies for urban and regional development. The advancement of these strategies is being driven by the growing interest in using decentralized generation technologies that rely on renewable sources. Moreover, there is a recognized necessity for conducting cross-sectoral evaluations combining various sectors such as industry, households, and transportation. The decision-making instruments discussed earlier are part of this kind of comprehensive energy planning framework : energy planning in cities and territories goes through a phase of design modeling.
The assessment of energy scenarios in urban planning involves heavy computational demands. One solution to this problem involves reducing the number of buildings by using Machine Learning (ML) techniques to cluster city buildings Torabi Moghadam et al. (2019). This approach simplifies the building stock by employing archetypes, which represent average reconstructed buildings or sample buildings. To go even further and to incorporate interactions across the community’s buildings, the concept of an “energy hub” has emerged, introducing a new layer of complexity to energy modeling. An energy hub serves as a focal point for the production, consumption, storage, and conversion of multiple energy carriers. While a single residential building can be considered as a micro-hub, a cluster of these micro-hubs forms a macro hub, often referred to as a district or a neighborhood (Middelhauve, n.d.). However, a precise definition of a neighborhood and especially the appropriate scale to consider has yet to be clarified.
Furthermore, neighborhoods can, in turn, be clustered in order to extract neighborhood archetypes (average reconstructed or sample) to reduce even more the computational complexity of modeling energy system at a city level (Loustau et al. 2023). Loustau et al. clustered neighborhoods of Geneva according to their energetic characteristics, i.e. the energy demands (heating, hot water, electrical) and the endogenous resources (solar, biomass, geothermal) and finally obtain 4 typical neighborhoods for the whole Canton of Geneva.
2.2 Local energy market
Traditional centralized energy systems can be characterized by a large number of customers located within a wide area. Energy is supplied by large power plants that operate according to a centralized coordination mechanism. Decentralized energy systems, on the other hand consist of small-scale energy generators that are placed in the same location with an energy consumption (Alanne and Saari 2006). Energy communities can help in ensuring this reliable supply.
Local energy markets can reduce the need for expensive and inefficient energy transportation with substantial losses (Jimeno et al. 2011) by satisfying demand from local energy resources. Furthermore, the latency for managing congestion and distribution faults can be decreased (Kuznetsova et al. 2014). These communities strengthen the local community in terms of self-sufficiency and provide the possibility of energy cost reduction. Local transactions keep profits within the community and encourage reinvestment in additional renewable generation (Koirala et al. 2016).
In Switzerland, the building scale is still strongly institutionalized and appears to be the reference scale but the neighborhood scale is more and more recognized as a fundamental scale. At the building scale, there exist two legal possibilities for households inside a same building to share and trade electricity in Switzerland : CA for Comunauté d’Autoconsommation in French, meaning self-consumption community in English; and RCP simple. These two models aim to promote the use of renewable energy sources and increase energy self-sufficiency at the building level. Figures 2.1 and 2.2 show schemes of a CA and a RCP simple
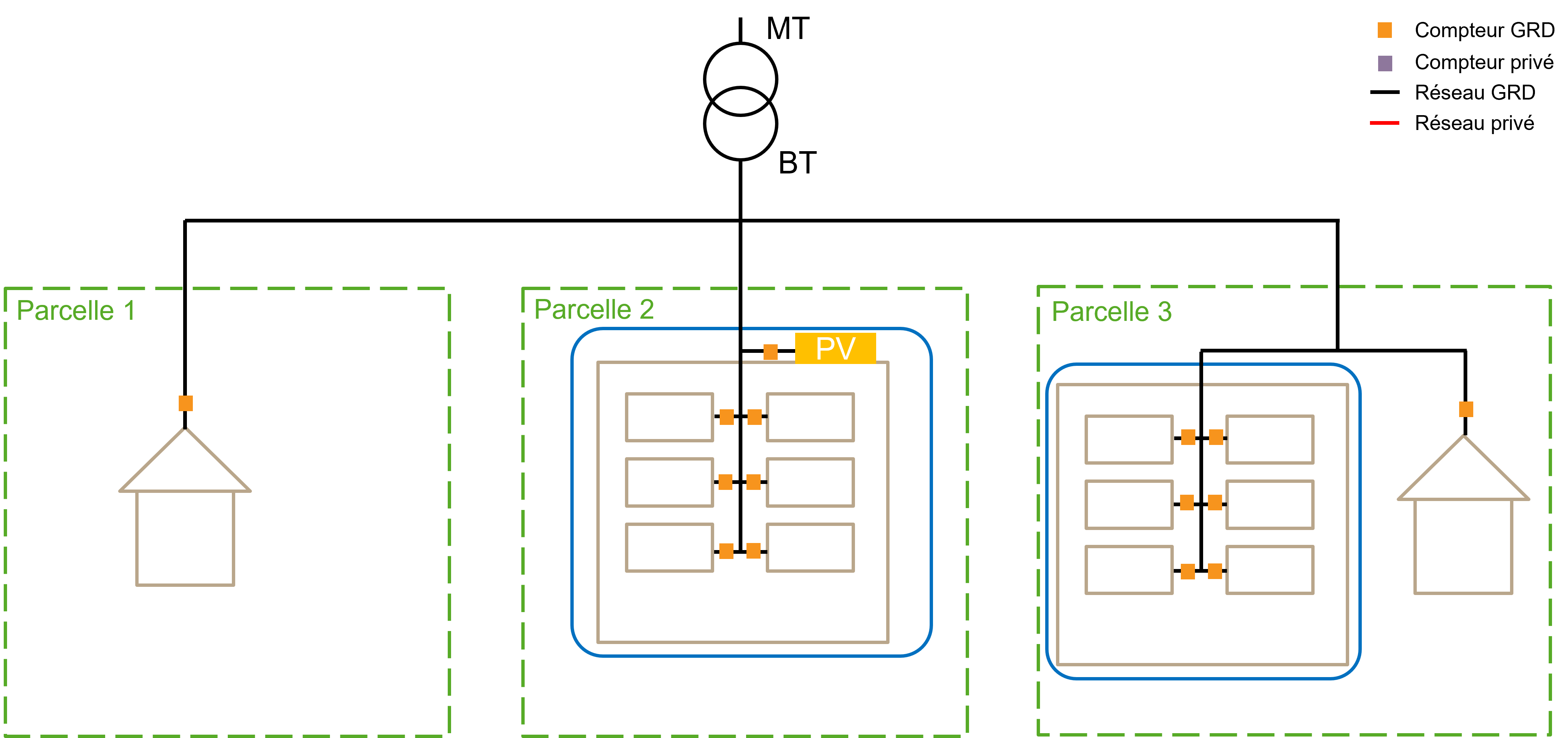
Figure 2.1: Representation of a CA
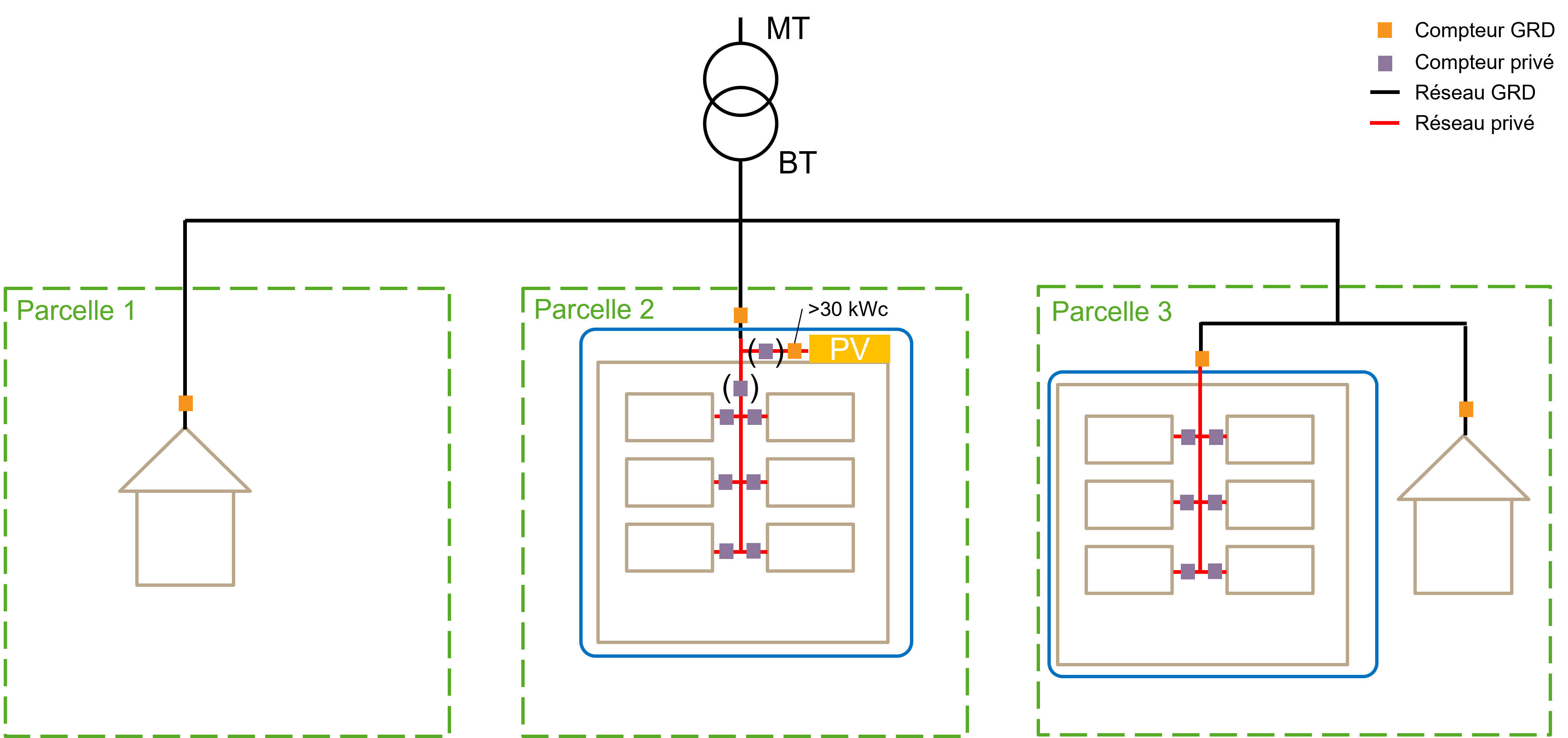
Figure 2.2: Representation of a RCP simple
In a CA, households gather to consume locally generated electricity from renewable vector such as PV panels own by one or more of its members. Trading rules are establish through agreement among its members. This allows for potential cost savings due to self-consumption and lower grid reliance. Each household is however still considered as one end-user for the DSO. In a RCP simple, households share and trade locally produced electricity in the same way but are no longer considered as end-users for the DSO : It is the whole RCP, thus the entire building which is considered as the end-user. The billing for each household is made within the RCP members and the electricity meters of each household becomes private (no longer owned by the DSO). It can be seen as a group of consumers who collaboratively organizes to optimize their energy consumption and potentially derive benefits from economies of scale.
As explain previously, the only way to self-consume at the neighborhood scale is through the implementation of RCP microgrid that enable to connect multiple buildings together thanks to a private network. In this case the whole community is still seen as one end-user for the DSO. However installing a private, new network is not really convenient for some neighborhoods. Figure 2.3 shows a scheme of a RCP microgrid. It appears thus important to find new legal way of self-consuming, sharing and trading energy at the neighborhood level. Some experimentation are conducted in order to identify the leverage that this scale could bring for the national energy transition as for instance the Quartierstrom project.
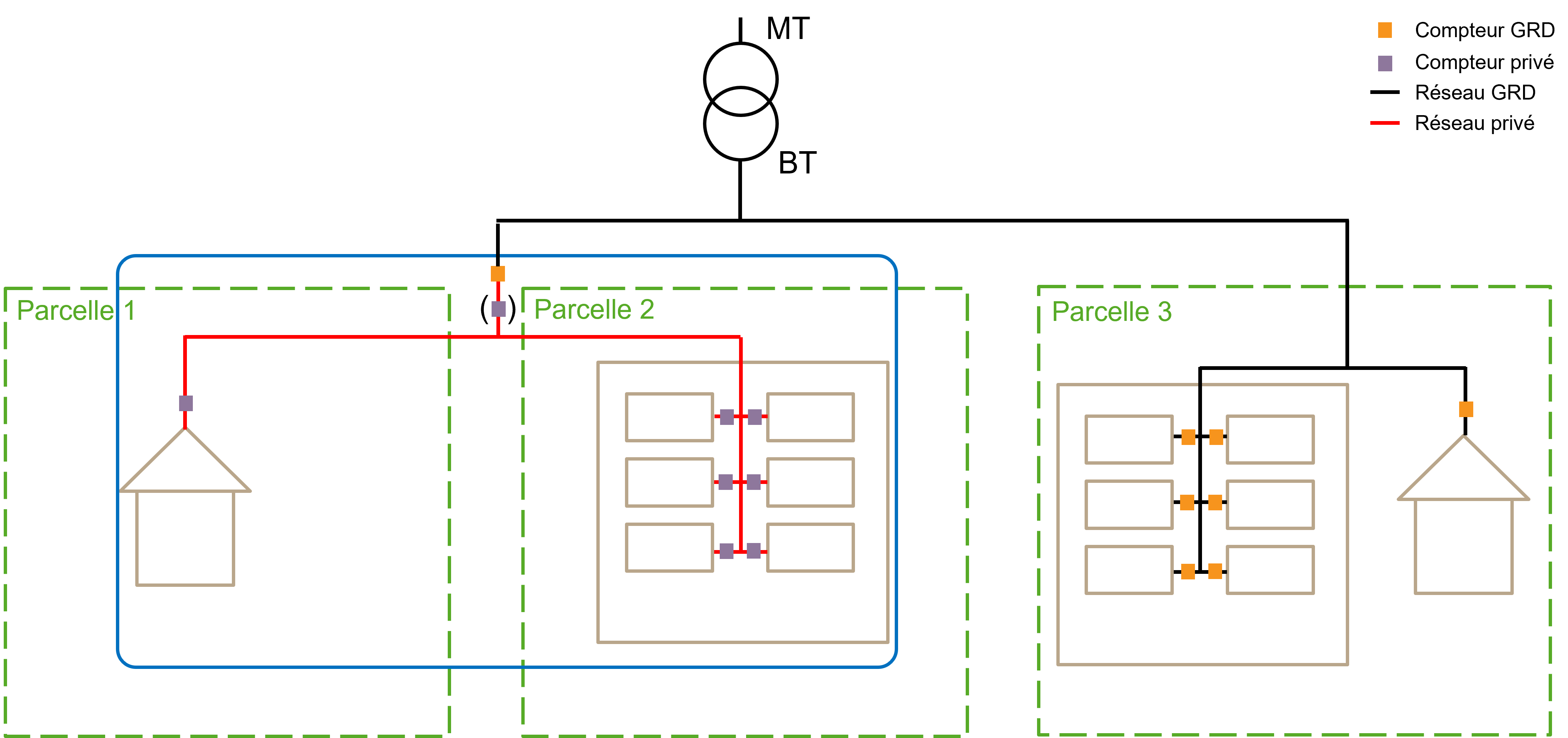
Figure 2.3: Representation of a RCP microgrid
The Quartierstrom project (Office fédéral de l’énergie, n.d.) has been supported within the flagship program by the Swiss Federal Office of Energy and examines a real-world peer-to-peer (P2P) energy market. In close cooperation with the local utility company EW Walenstadt, the project was to implement a P2P energy market based on blockchain technology with 37 participating households and a retirement home in the town of Walenstadt (Canton of St. Gallen). Prosumer households, who already owned PV panels, could sell their excess solar production directly to neighboring households without interference or reliance on a trusted third party but using the network of the DSO. If energy demand or supply could not be traded within the local community, the local utility provider, EW Walenstadt, served as backup to fill excess capacities at fixed tariffs. As the network of the DSO was used to exchange energy between the members, a local distribution charge was imposed on every excahnges inside the neighborhood. This allowed the DSO to capture value from the neighborhood internal flows.
During the one-year field phase of the project, the solar panels in the neighborhood have produced over 250 MWh and the participating households have consumed almost 470 MWh, 70 MWh were sold from household to household within the community. Local energy trading almost doubled the community self-sufficiency rate (from a 21% to 39%) and self-consumption rate (from 34% to 62%). However, although many participants stated an appreciation for their ability to directly influence the price they paid/received for local electricity, towards the end of the project, a majority of them expressed a preference for a system with a higher degree of automation.
European countries also try to restructure their business model of energy supply. France for instance, has implemented a special distribution charge (timbre in french) system for self-consumption communities using the public grid : collective self-consumption is made possible if the consumers and/or producers of specific area gather in a single moral entity. The energy price of electricity is negotiated between each members but the distribution charge is fixed to a special official price. The DSO is responsible for carrying out metering activities for members (producers and consumers) in order to establish appropriate billing : it monitors the auto-consumed part, the volume of import electricity from the grid for consumers. In this case, members can gather only if the distance between each actor is less than 2 km. Distance between actors is thus the key criteria for being part to this kind of community. They also need to be connected to the Low Voltage (LV) network but not necessarily to the same Medium Voltage/Low Voltage (MV/LV) transformer.
Austria also proposes already a model of collective self-consumption at levels 7 and even 5 by gathering consumers, producers and storage solution into a single legal entity. Figure 2.4 shows the representation of the 7 voltage levels of the energy transmission and distribution networks.
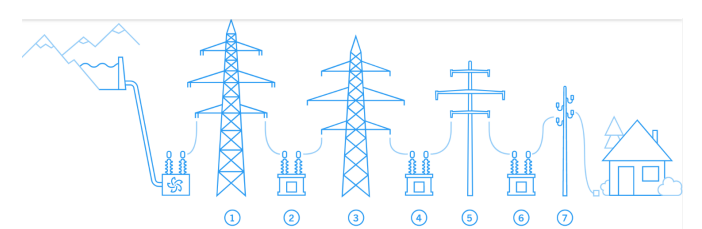
Figure 2.4: Representation of the 7 voltage levels of the enrgy transmission and distribution networks
In this case, distance between actors, which can be seen as a constraint for being part to a self-consumption community is no longer a criteria. It is thus possible for a municipality or a DSO to create a collective self-consumption community and to group together local renewable energies, consumers and future consumers in this community, which then becomes an energy hub. Figure 2.5 shows a scheme of the implementation of such community in Austria.
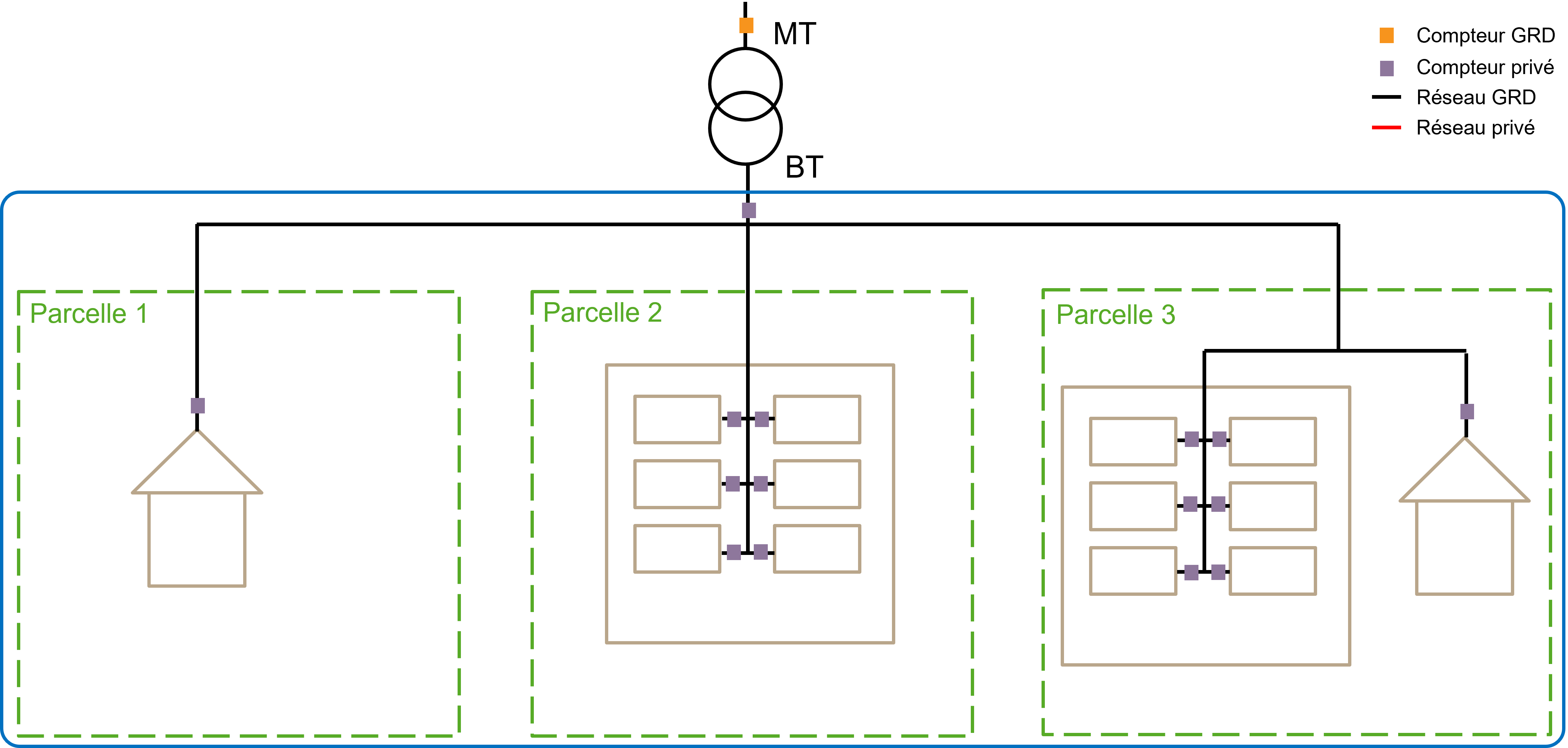
Figure 2.5: Renewable energy community in Austria
What would even more interesting for the DSO is keep public electricity meters so that it knows what happen inside the energy community and it could take care of metering activities for members (producers and consumers) in order to establish appropriate billing. Figure 2.6 shows this ideal energy community implementation.
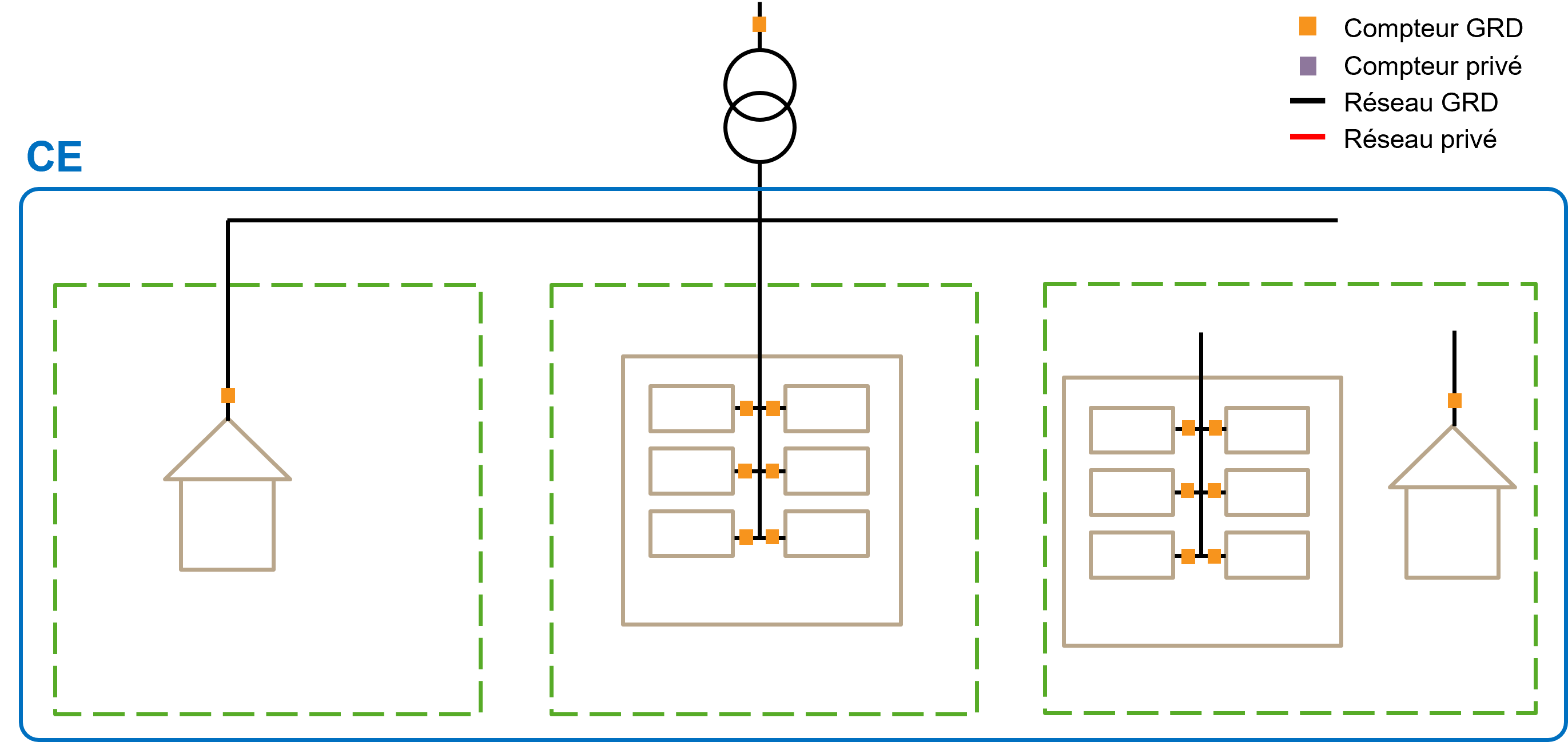
Figure 2.6: Ideal energy community in Switzerland